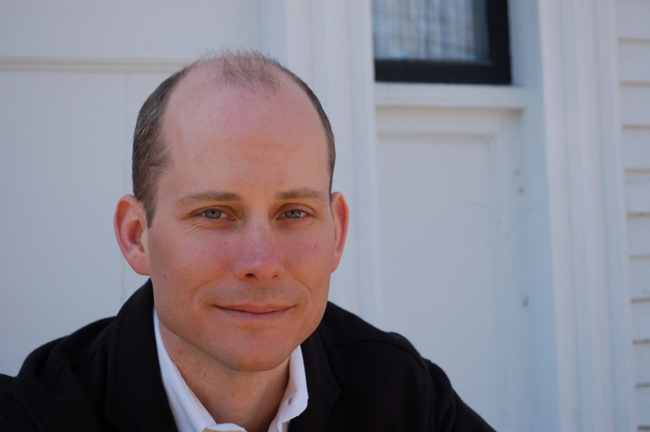
While artificial intelligence is infiltrating most areas of our lives and work, many people see it as tool for and of science – largely useful to programmers and data scientists, certainly not for English majors. Andrew Piper, a Faculty of Arts professor in Languages, Literatures and Cultures, is helping bridge the gap between AI research and the humanities, with his focus on data science in literature. Using machine learning to analyze texts, he can hone in on bias, data selection and answer limitless questions about the ways we understand and analyze literature, film and other cultural artifacts.
Piper recently participated in McGill’s AI For Social Good Summer Lab, during which he spoke to the cohort of exclusively female students about using AI to detect gender bias in book reviews, a project he has been working on with students in txtLAB. We sat down with Professor Piper to find out how he got into data science in the first place, and where he sees the marriage of AI and the Arts going in the future.
Could you tell us how you got involved with AI and data science?
Four or five years ago I ended up with a Fellowship from the Mellon Foundation, called the New Directions Fellowship. It was designed so that profs can train themselves in some area. My area was in data science, although at the time it wasn’t quite as common a term as it is now.
In the process of learning how data, computation, and statistics work, the goal was to apply it to my field of study – literary studies. It’s been a really steep learning curve, and a lot of it is coming through good collaborations with colleagues here and at other universities. That’s where things started and they’ve snowballed ever since.
Did you have an idea that you would end up with the focus that you have now, looking at gender bias, or bias in literature?
I don’t think I had a lot of expectations when I started. We knew we had a problem in our field of way too many documents. I gradually realized there was a whole field developing that was trying to approximate how we read, and that’s a really interesting question because what we study in our field is reading.
I was really trying to understand how a computer models a document, or a text. What were the assumptions built into that? How much does that align with where we’ve been? One of the challenges of applying this to literary studies is depending on how you count, we are between two and three thousand years old. Biostats… 20 years? We’re a couple orders of magnitude older than that, so we have enormously long traditions of practices, norms, expectations, beliefs. The question was how to align these things with a very different genealogy. Over time I gradually figured out what tools were better at doing or detecting. Biases are obviously a classic, because they’re just about patterns of usage, and they have very distinct social applications.
Is that important to you? Is using data science to understand our biases and doing something socially useful a part of the driving force behind your work?
It was another thing that got me excited about this work that I hadn’t seen before in my research – social relevance. You get to a certain point in your career where those questions may be more or less important, but where I am now it feels increasingly urgent.
One of the things we’re beginning to look more into is not just the social biases that are encoded in language and texts and creativity, but also where we’re getting things when we study them. A lot of literary studies or what we think about in the history of literature is really from a tiny, select, and elite corpus and community, and that perpetuates a certain mentality.
If you run through an English Major’s system, it’s really hierarchical and focused on taste, quality and value. What computation brings to the field is pushback to that, and asking ‘What about everything else?’ I suspect a lot of the reason we didn’t look out for it is because we just didn’t know how. The nice thing is we now have tools where we can look at hundreds of thousands of fan-fiction documents, or user-generated self-publishing platforms. It’s not just questions of bias but also the bias in how we select things.
How did you decide to look at gender bias in book reports, given the breadth of choice in the field?
The gender bias project was driven by a student, actually. We had done some research on this with a colleague at another university, and she was reading about it and told me of my ethical responsibility to do something about this problem.
It was a crystallizing moment for me, because I had never before thought of advocacy in my research. In the past I worked with 18th century German literature and there’s just not much to advocate for there. I’ve realized in the social sciences they have this whole mediating mechanism about what we do with our research, but then they have policy that looks at how to turn research into action.
I think that’s going to be a new and interesting area for cultural studies. We can analyze these things, but how do you change people’s behaviour? How do you get editors to actually stop choosing more books by men, or stop choosing more books about certain topics when they review books by women? I don’t know the answer to that yet but I think it’s a really interesting problem to solve.
So what led you to get involved with the AI summer lab at McGill then? I noticed you were the only session that involved an Arts professor.
That was an initiative on Angelique Manella’s office’s part (McGill Office of Innovation), and it came out of this idea of popular conceptions of AI can be very skewed and oriented towards certain applications and certain flaws and problems. I think her goal is to restart the conversation around social good – can we use AI for something that seems more beneficial and inclusive? I think she came across the project we had been doing on gender bias in book reviews, and kind of connected the dots, and I was very happy that she did.
When the opportunity came along, I jumped in because it was exactly the type of thing I wanted to participate in, with an audience I was particularly interested in speaking to. It was very clear that she was also trying to address gender imbalances within the industry or field, which is great.
So how are the students who are involved with your lab typically coming to you? Finding out by word of mouth, are they in your classes…?
A little bit of both. Some is definitely word of mouth: a student who’s taken a class will have a friend who’s in computer science, or affiliated with another lab. I teach an introduction to text and data mining every fall to undergrads. The assumption is that if you want to work in the lab you have taken that class or are enrolled in it, so you have some of the basic skills needed to do the work.
I’m also seeing, by collaborating with colleagues and other parts of the university, that there are students who are missing a certain dimension of their interests from the lab they’re associated with, usually in more traditional Computer Science backgrounds and environments.
With my lab, students who are interested in both CS and the arts and humanities can put both together. I think it hits a certain type of student, who’s really not finding that connection on campus, and they get really excited about it. It gets good retention; they get involved, stay involved, stick around, but we don’t have a big population. The combination of students who are interested in computation and literature and culture is not huge right now.
How many is ‘not huge’?
Well my 200-level intro class usually finishes with 18-20 students. I start with a lot more, around 60 students, but they get scared off. It’s not true of my other classes – It’s not just because I’m a terrible teacher [laughs], it’s also the subject matter. It is tricky right now to find students who are comfortable in both domains. That’s a bigger, extrinsic thing to work on, and I don’t really know how to address it. They completely push this idea that there’s the science folks over there, the humanities and arts folks over here. [We should be] creating forums, spaces, initiatives where people just don’t continue to think that way. That’s something I’m really invested in.
Have you had any students who you’ve had that light go on? That they realized it was like learning a language, rather than being just about math?
Yes, and it’s an awesome experience to watch. You can see it when all of a sudden, they realize that taking one book and using it as an example for every book ever written, is a terrible idea. They see all the problems with it, and that [data science] allows them to think more critically about those choices and how to analyze this material. And yes, there’s a lot of challenges on how to get a machine to reflect analytically on what is trying to be understood, but it’s like [learning] a language.
Are there other parts of the humanities that you can think of that this type of research would also be applicable to?
I think wherever there is language and complexity, it’s relevant. We’re using it to analyze fan-fiction, but we’re also using it to analyze TV screenplays, so how people talk in movies and television. We can use it also to analyze spatial relationships in these shows, so where are these things set, what are the biases in terms of domestic setting versus workplace settings? They allow you to do all this kind of critical analysis of culture – how it’s made, how it’s produced. There’s not a corner of humanities where that’s not a problem.
If you’re looking at history, you inevitably have more documents than you could ever read that are available to you to study, so what kind of models do you need to build in order to make assessments about what’s going on in the past? That’s true in anthropology, communication studies, media studies, literary studies…. We all have the same problems. And it’s a new way of trying to tackle those things.
One of my goals is to see this become more interdisciplinary, not just between computer science and the humanities, but more general across the humanities. It’s a shared platform for a shared problem, and it’s really interesting to talk to political science majors about how they analyze parliamentary transcripts, and the types of questions they ask about voter behaviour, or belief systems, which are expressed through language. In the same way we think about novels and authorial creativity, it’s being expressed through language. The underlying models and the questions you can bring are very similar, and it lends itself to more cross-talk.