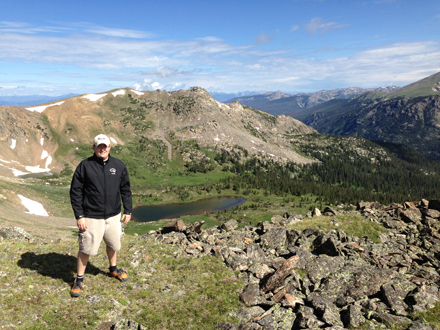
By Sean Grogan
One of the more exciting developments in the last few decades in the mining industry has been the use of mathematical models, geo-statistics and other management science and statistical methods to create tools to be used in the decision making process for engineers, managers and shareholders.
Traditionally, mine plans were done iteratively, making adjustments to plans little by little until an “optimal” schedule was developed. Optimal is in quotation marks because it cannot be necessarily proven to be mathematically optimal. Mine plans done with this approach may be developed unsystematically and with biases. Potential errors may propagate through an unsystematic way of developing schedules.
But by using management science techniques, it is possible to have an unbiased, systematic approach to the mine scheduling process. While no model is perfect, the fact that management science approaches problems in a systematic, unbiased way can allow engineers to understand the process, its advantages and limitations, and thus make even better decisions for the company.
This past summer I had the privilege of working in the Mine Optimization Department at Newmont Mining Corporation in Greenwood Village, Colorado. Living in Colorado was a great change in pace for me. As an avid cyclist and outdoorsman, it was great to explore the larger mountains the west had to offer.
My human resources manager referred to Denver as a ‘cycling haven,’ which may have been an understatement. It was not difficult to route my way to and from work every morning away from busy roadways. However, the outdoors lifestyle is not for the faint of heart. I went to Colorado believing the altitude change would have had little effect on my athletic endeavours. I thought it would take me a day or two to adjust and I could live my life the way I had in the great Northeast. However, it took me almost month to get to the point where I could complete a run without having to stop to catch my breath every 10 minutes. It looked forward to my return to sea level however because, according to claims, I would be able to run forever.
This work term was a departure from previous work terms where I was a heavy equipment operator and had done short-range planning in Northern Alberta. While the differences are apparent (field work versus sitting in front of a computer all day doing computer programming), they are similar in the regard that they both were outside my comfort zone. Having grown up in the suburbs of Jersey, operating a large vehicle, one as big as the house I grew up in was a challenge both physically and mentally. In the latter I had changed roles from my formal education as a mining engineer trained to work in the field to being a computer scientist.
Those who have earned a B.Com in Operations Management or Operations Research will recognize the tools and techniques I had the opportunity to learn and work with on my work term. I was predominantly working with a technique called Mixed Integer Programming to set up a mathematical model that would attempt to make decisions to maximize the value of a project, subject to several constraints.
Mixed Integer Programming can allow for great flexibility and allow for a wide number of decision variables to be programmed. An engineer can set up a model which is large enough to have decisions for a specific mining block from mining to where a company may send the material (smelters, steel mills, etc.). However, with larger models (more decision variables) comes longer solving times. A lot of research is going into both decreasing solving time and/or decreasing the number of decisions to be made. This is where engineers who create the models will know their model’s limitations.
In addition to using the mathematical models to try to schedule an open pit mine, I had developed other techniques to assist in the speed of solving for an open pit mine. One such technique was attempting to group block together by attribute similarities, such as grade and distance.
Because of the relatively recent development of Mixed Integer Programming applications in the mining industry (late 60’s), techniques and applications are in its infancy. There are still very crude models with regards to underground mining and modelling, the modelling of stockpiles, and the modelling of a transition between open pit and underground mine. Other applications will present themselves with time. Such applications can include the use of fleet selection in a mine.
With the approaching retirement of the ‘baby boomer’ mining engineers, I am still presented with opportunities in the traditional mine planning and mine management roles. My generation may be the last generation of mining engineers to graduate and enter the work force without a deep appreciation, understanding and training with the management science approach to mine planning. There will come a day where advanced computer science classes and other courses in mixed integer mine planning will become common.
As I wrote earlier, there are many problems to still be solved. Unfortunately, people with the skill set to solve these said problems are often lured away by companies such as Google, Microsoft, IBM rather than to companies or schools with mine optimization programs (Such as Newmont, BHP, McGill, and U of A). As a pitch to computer scientists and operations management majors, there is a need for your skills in the industry. Many people have told me they got into mining was because of the down-to-earth nature of the people in the industry (The puns!). For my fellow miners, these skills are going to become paramount in the coming years.
Sean Grogan is in his fourth and final year as a mining student in McGill’s Mining Co-Op Program.